Agentic AI vs. Traditional Automation: What’s the Difference?
What if your AI wasn't just answering questions, but asking the right ones? What if it didn't just follow instructions, but understood your intentions and helped achieve your goals even when plans go sideways? Traditional AI is like having a highly skilled assistant who excels at specific tasks - writing emails, [...] The post Agentic AI vs. Traditional Automation: What’s the Difference? appeared first on AutomationEdge.
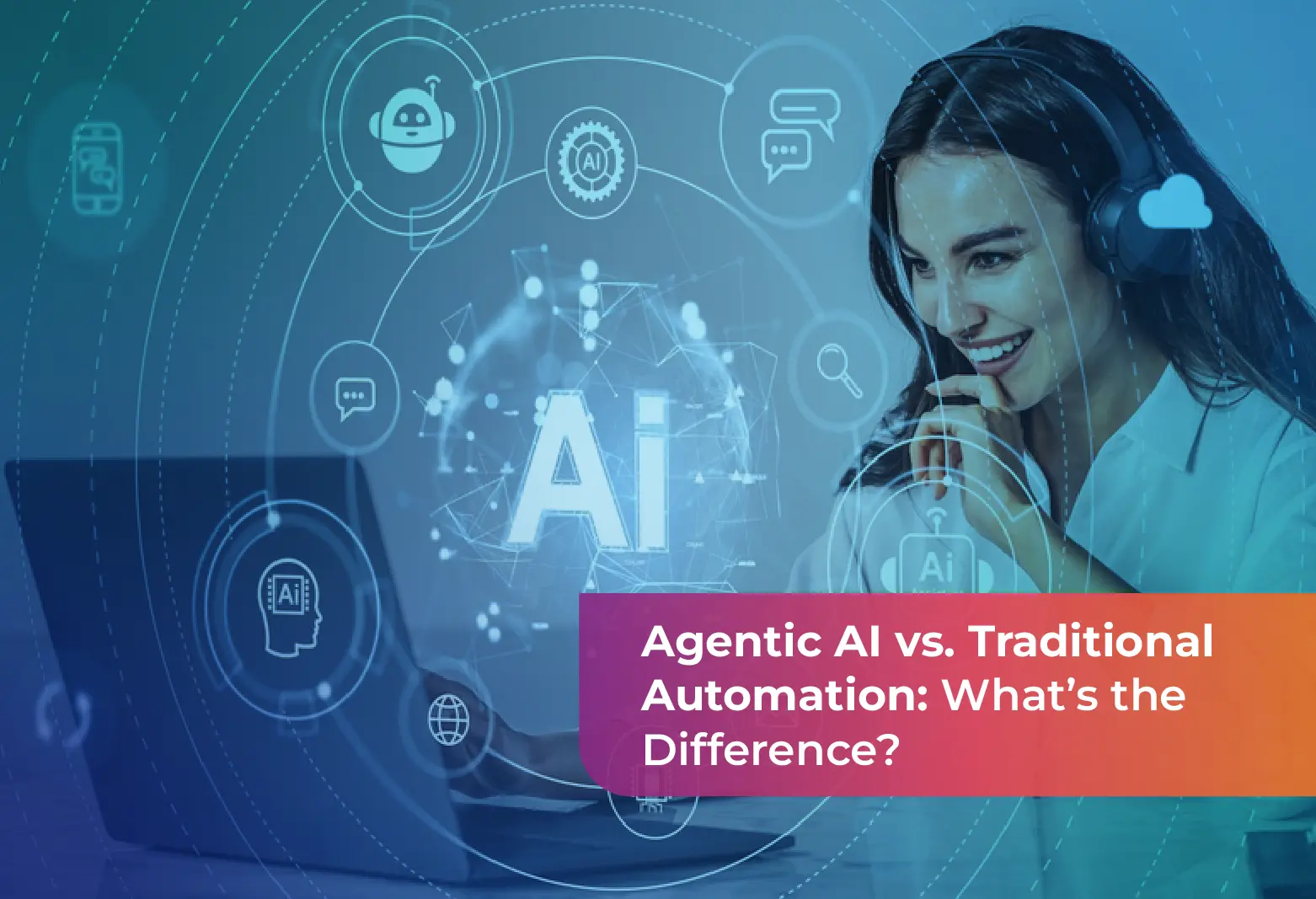
What if your AI wasn’t just answering questions, but asking the right ones? What if it didn’t just follow instructions, but understood your intentions and helped achieve your goals even when plans go sideways?
Traditional AI is like having a highly skilled assistant who excels at specific tasks – writing emails, analyzing data, or generating images. But imagine instead having a proactive partner who not only completes tasks but anticipates problems, adapts to change, understands context, takes initiative to learn and evolve. The shift from Traditional to Agentic AI isn’t just about better task completion – it’s about moving from reactive tools to proactive partners in achieving our goals. In today’s fast-paced, complex business environment, we need AI that doesn’t just process information but helps navigate uncertainty and drive results.
Traditional AI is like a highly skilled GPS that efficiently calculates the best route from point A to B based on pre-existing map data. While impressive, it’s fundamentally reactive to your input. Agentic AI, on the other hand, is more like a seasoned personal driver who not only knows the route but actively monitors traffic updates, remembers your preference for avoiding highways, notices you’re running late for a meeting, and proactively suggests leaving through the back roads while sending a courtesy message to update your meeting participants. The key difference is that Traditional AI follows a script, while Agentic AI thinks ahead, adapts to changing conditions, and takes initiative to help achieve your broader goals.
Unlike traditional AI models that follow predefined rules or Generative AI that focuses on creating new content, Agentic AI emphasizes goal-oriented behavior and adaptive decision-making. In banking and insurance, AI agents can initiate loan applications, update accounts, assess credit risk, and even automate claims processing. They continuously learn from interactions, allowing for a more personalized and efficient customer experience. This reduces the likelihood of errors and frees up human employees to focus on higher-level, strategic decision-making. Financial institutions enhance operational agility and customer satisfaction while reducing manual workloads.
Listed below is a comparison between Agentic AI and Traditional Automation
Aspect | Agentic AI | Traditional Automation |
Core Features | Adaptive, intelligent decision-making capabilities based on context and data analysis | Rule-based systems that perform pre-programmed tasks |
Functions | Automates complex workflows like customer profiling, fraud detection, and financial planning | Automates repetitive tasks like data entry, transaction processing, and report generation |
Learning Capabilities | Learns from data and feedback, continuously improving over time | No learning or improvement over time; static once deployed |
Customization | Highly customizable with the ability to create agent templates and adapt to specific organizational needs | Customization is limited to predefined workflows and rules
|
Scalability | Scales operations without compromising on decision quality; ideal for growing volumes of data | Scales based on repetitive task automation but lacks the ability to handle complex decision-making |
Integration | Seamlessly integrates with various BFSI systems like CRMs, core banking platforms, and analytics tools | Requires manual integration and may face limitations in working across diverse systems |
Efficiency | Increases efficiency by automating both simple and complex decision-making tasks in real-time | Increases efficiency only in rule-based, repetitive processes |
Customer Experience | Provides personalized financial recommendations, real-time support, and proactive insights | Limited to automated responses for common requests, with minimal personalization |
Fraud Detection | Uses predictive analytics and pattern recognition to proactively identify potential fraud | Detects fraud based on predefined rules; less effective against evolving threats |
Support | Continuous support through AI-driven insights and decision-making | Basic support through predefined workflows and task automation |
Take for example, a small business owner, Sarah, applies for a $50,000 business expansion loan. She runs a seasonal beach equipment rental company with irregular income patterns and has recently diversified into offering surfing lessons.
Process | Traditional Approach | Agentic AI |
Data collection | -Uses fixed online form with predetermined fields
-Requires standard documents: last 3 years’ tax returns, bank statements, business registration -Rejects incomplete applications automatically |
-Engages in conversational interface to understand business context
-Dynamically adjusts required documentation based on business type -Proactively requests relevant seasonal business information -Suggests additional helpful documentation Sarah could provide |
Processing | -Applies rigid rule-based criteria:
-Flags applications not meeting standard criteria for manual review -Cannot process unconventional income patterns |
Analyzes broader context:
-Adapts assessment criteria to business model -Identifies patterns in seasonal cash flow |
Decision making | -Binary outcome based on predefined thresholds
-No consideration of business context -Generates standard rejection/approval letter -Routes complex cases to human underwriters |
-Holistic evaluation considering multiple factors:
-Generates customized loan terms aligned with cash flow |
Outcome | Sarah’s application is automatically rejected due to:
-Irregular income patterns don’t fit standard assessment models -Recent business diversification viewed as risk factor -Seasonal nature of business triggers risk flags |
The AI approves the loan with customized terms:
-Flexible repayment schedule aligned with seasonal income -Higher payments during peak season, lower in off-season -Includes specific provisions for the surfing lesson expansion -Offers business advice on potential revenue optimization |
AI agents are designed to integrate seamlessly with existing platforms, making it easy for companies to adopt this technology without overhauling their systems. These agents utilize advanced natural language processing and machine learning algorithms to understand and respond to customer inquiries, automate routine tasks, and optimize workflows. By continuously learning from each interaction, AI agents become more accurate and efficient over time, providing increasingly valuable insights and services.
Agentic AI offers a more dynamic and intelligent solution for the BFSI industry, capable of managing complex tasks, while traditional automation focuses on simplifying and automating repetitive, rule-based tasks.
The post Agentic AI vs. Traditional Automation: What’s the Difference? appeared first on AutomationEdge.